Augustin Marty, CEO at Deepomatic, outlines how AI emerges as the ultimate ally for fibre optic networks in 2025. Learn about the key challenges and solutions for automation, predictive maintenance, and more.
We are currently in a place where the fibre optic communications industry is at something of a transformative crossroads. The convergence of a number of market factors has created an environment where AI adoption in fibre networks isn't just advantageous, it’s actually now becoming essential for survival. From my perspective, I've observed how several factors have aligned to make this year particularly significant for AI implementation in fibre broadband networks.The catalyst for this transformation stems from six key market conditions that have perfectly aligned.
Expanding fibre networks urgently, without burning costs
First and foremost, we're seeing an unprecedented demand for ultra-reliable connectivity. The continuous growth in video streaming, gaming, and remote work has created an urgent need to expand and upgrade fibre optic networks at a pace we've never seen before.
Secondly, rising operational costs, particularly in maintaining and scaling fibre networks, are pushing wholesale carriers and ISPs toward AI adoption. To achieve sustainable growth, operators must automate crucial processes such as deployment planning, fault detection during fieldwork, and network traffic management. With such a rapid expansion, manual processes are no longer viable - they are too time-consuming, resource-intensive, and hinder the ability to design, deploy and manage networks at speed while maintaining high-quality standards.
Government investment and regulatory pressure
A third factor is the level of government investment in broadband infrastructure. In Germany's, the 2025 Gigabit Funding programme is making €1.2bn available for fibre broadband infrastructure projects. Recently, the The Catalan government in Spain has announced intentions to invest EUR 91.5 million in expanding the publicly owned fibre optic network.
This investment comes alongside increased regulatory oversight - our fourth key factor. Governments aren't just providing funding; they're mandating service quality improvements. In the UK, for instance, Ofcom requires ISPs to meet strict quality-of-service (QoS) standards and report on network performance, mirroring similar requirements in France.
Market dynamics and workforce challenges
The fifth factor stems from market dynamics driven by the aforementioned boom in funding. In the last five years, we've seen the inception of numerous new players, particularly in the US and UK, where alternative providers (altnets) are aggressively deploying fibre in underserved areas. This fierce competition is forcing all operators to differentiate themselves in saturated markets by rolling out sustainable infrastructures, building reliable systems of record, and offering outstanding quality of service to their subscribers.
Perhaps most importantly, our sixth factor is the significant workforce deficit facing the telecom industry. Operators face immense pressure to deploy fibre networks quickly while grappling with a shortage of experienced field staff. Senior employees are retiring, and recruitment of skilled replacements is challenging. This shortage, combined with the need for speed, jeopardises quality. The primary challenge lies in delivering high-quality work at the required pace with the available workforce.
The AI solution: transforming network lifecycle management
In response to these challenges, operators are actively seeking AI solutions across three main areas of the network lifecycle:
In network planning and design, AI-driven tools are being used to help operators plan fibre routes, considering cost, efficiency, and feasibility in both urban and rural environments. AI-powered GIS platforms can now suggest the most cost-effective and feasible network paths, while digital twins allow operators to test designs virtually before deployment.
In fibre construction, AI can be used to allocate resources based on worker availability, skill level, and location. It helps track deployment timelines, flag delays, and recommend actions to keep projects on schedule. On top of this, AI can predict project costs by analysing past projects, current market rates, and material availability. When it comes to quality control, AI, and more specifically, computer vision, can analyse photos taken by field workers during all construction operations. Live feedback is provided to inform them in case of error or oversight, to allow them to correct the anomaly before leaving the site. By collecting data about the assets that are installed or built, AI feeds the operator’s digital twin, ensuring that it is consistent with the network’s design plan.
During the fibre deployment phase, operators can also rely on AI to automate their quality control processes, and make onsite or remote audits a thing of the past. Not wasting time in fire construction and deployment is essential, and this is a key value of real-time quality control, as it reduces revisits. This way, operators no longer face expiring wayleaves which can put a hard stop to their activity for a couple of weeks.
Then there is the maintenance side, which can be incredibly time-consuming, especially when it comes to buried or large-scale infrastructures. Operators historically planned maintenance operations when equipment was experiencing functioning issues, or at a specific frequency, without knowing if equipment needed it, and where to go in priority.
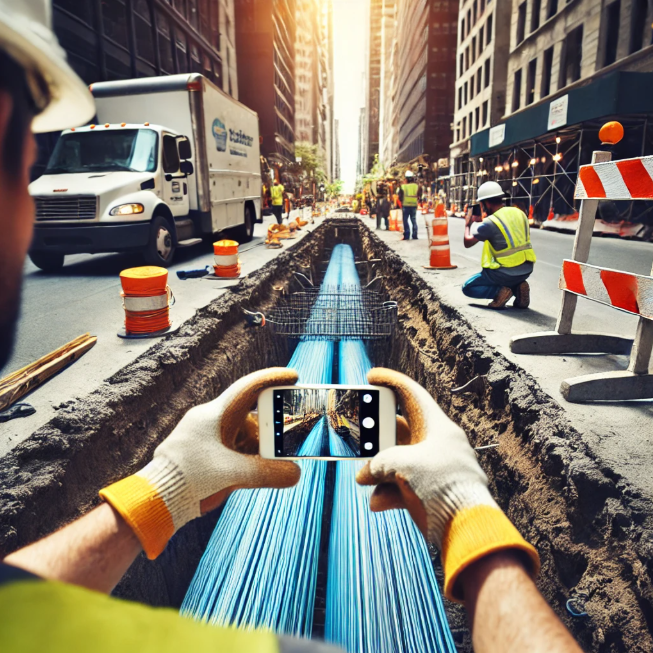
Operators are actively seeking AI solutions across three main areas of the network lifecycle
Now, with AI, operators can monitor real-time equipment condition, in order to know when and where to schedule maintenance in priority. They can also analyse historical and real-time performance metrics, such as signal strength and asset degradation, to predict equipment failures before they occur. AI can flag segments likely to degrade which need maintenance, to avoid unexpected downtime.
Proven ROI and success stories
The benefits of AI implementation are no longer theoretical. We're seeing real results across the industry. Swisscom, for instance, tripled its quality control capacity while increasing the pace of network deployment, and all without additional hiring. Similarly, CityFibre in the UK reduced manual review time from 45 minutes-per-operation to mere seconds, thanks to AI-driven automation.
We recently commissioned Forrester Consulting to conduct a Total Economic Impact™ (TEI) study to examine the costs, benefits and ROI of our AI platform. It revealed that AI-driven quality control automation can deliver more than 420% ROI within three years. On average, our clients reduce revisits from 10% to just 1%, representing millions in savings. They also achieve significant cost reductions by reducing on-site audits from 10% of operations to just 1%.
AI: The challenges and perceptions
Of course, AI implementation in network infrastructure also comes with its share of challenges. Data security and privacy are incredibly important when handling sensitive network information, so robust encryption and anonymisation protocols are required to protect both operator and customer data. AI systems should also be trained on diverse, high-quality data to avoid biases that could lead to skewed network optimisation decisions.
Then there is the perception that AI will lead to job displacement. Interestingly, we're actually seeing AI create new opportunities in data analysis and AI system management, complementing rather than replacing human expertise. The real challenge lies in its integration. Merging AI systems with existing network infrastructure can require careful planning and expertise to ensure seamless operation without disrupting current services. However, these challenges shouldn't deter adoption; instead, they should be taken into account to inform how we approach AI implementation thoughtfully and systematically.
Implementation advice for operators
For operators beginning their AI journey, I recommend starting with a clear business case. Identify specific objectives - whether that's reducing repeat visits, cutting quality control costs, having a clear representation of their network, or improving customer satisfaction.
I advise choosing an AI vendor with proven experience in your vertical and AI models tailored to your specific needs, to accelerate the deployment of your project
Quality of data for AI training is crucial. AI models require clean, structured data to function effectively, so operators must standardise their data collection methods to ensure consistency and accuracy. This standardisation is particularly important for field operations, where environmental conditions and technical requirements can vary significantly.
Equally important is change management. Success requires preparing all teams that will be impacted by AI implementation. This means raising awareness about the urgency of breaking free from previous processes, communicating changes and benefits for each role, organising comprehensive training sessions, and ensuring smooth user experiences to reduce adoption barriers.
Looking ahead
As we progress through 2025, the role of AI in fibre networks will only become more important. The combination of government investment, increasing competition, workforce challenges, and operational pressures has created an environment where AI adoption is no longer optional for operators seeking to maintain competitive advantage.
The future of fibre networks lies in intelligent automation, predictive maintenance, and data-driven decision-making. Those who embrace these technologies now will be best positioned to deliver the reliable, high-speed connectivity that our digital future demands. The question is no longer whether to implement AI, but how quickly operators can adapt to this new reality.